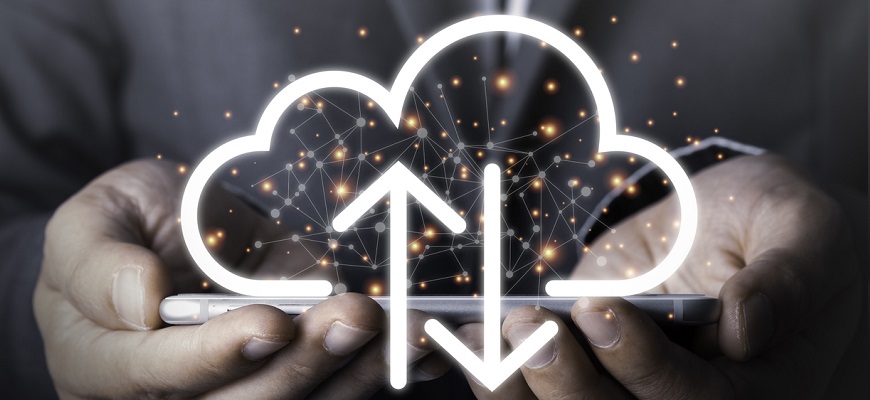
The COVID-19 crisis has drawn increased attention to government data, and to the ability to quickly link important data sets across diverse sources.
Certainly, there have been some disappointments in quality and timeliness of data, and the availability of data in electronic format. But in many cases the career public servants who have collected data for many years are only now seeing their important work gain public attention. Excellence in government data management is not new. Many of the best leaders and innovators in government data analytics have been refining their craft for over a decade, building success incrementally over time.
If the focus on data during the pandemic response and recovery period means that data will maintain its central role in policy making, now is a good time to examine not just the end result of having data available for policy makers, but how that data came to be available – the organizational and operational models for combining data sets into actionable insight for government decision-makers. In looking across data integration success cases in the United States and across the globe, we can generalize about the ways in which the work is led, managed and how the relationships are structured. The two models described here provide a way of thinking about the tradeoffs to be considered in establishing a new intergovernmental data sharing initiative.
Two main models. Two main models characterize how intergovernmental data sharing efforts are led and managed – one is primarily led and managed by employees who are inside government and the other is typically led at the policy level by government staff but at the operational level executed by collaborating third party intermediaries, such as a university, a think tank, a research organization or a data standards organization. In practice, there are often hybrids that draw upon characteristics of both models. Each model has its advantages and challenges.
While the two models are described separately, they represent a continuum. For example, a government led and managed data sharing effort may hire outside contractors to perform specific duties, like specialized research, but retain overall project leadership and control. Further, in the collaborative model, if the external data services provider is a university that is part of a public university system, then the operations are still using government resources. With this in mind, the models can be thought of as describing a spectrum of how responsibility is assigned and tasks executed.
To illustrate the difference between these two models, Allegheny County’s Department of Human Services data warehouse is led by public sector employees, is located on government office space and is staffed with a combination of public sector and contracted private sector employees. Given that leadership, location and much of the staffing is done by the public sector, this would be considered an example of an internal, government-led model. Expertise for predictive analytics modeling is often contracted out, as was done for development of both the risk model for child welfare and also for the recent homelessness services prioritization project. However, on balance, the ongoing work principally accomplished at a government office by government employees so this is considered a government-led model.
An excellent example of the collaborative model is the Census Bureau’s Administrative Data Research Facility (ADRF). This effort to bring powerful cloud-based computing power to federal employees, academics and state and local government is a partnership between the Census Bureau and the University of Chicago, the University of Maryland and New York University. Expert data scientists from these three academic institutions are jointly providing both computing power and related hosting, along with training and technical assistance to build capacity in government to perform advanced data analytics.
Example of government-led data sharing: Data Warehouse and Performance Dashboards: Boston, MA. The City of Boston created a consolidated data warehouse in 2019, which as of early 2020 held 330GB of data from across 31 city departments. This was the culmination of a multi-year effort that was among the signature accomplishments of the city’s former Chief Data Officer. This single source of data, along with a centralized mapping platform, makes analytics projects easier for the Citywide Analytics Team and also provides an easy point of access for all data analysts in city government. After creating the consolidated data warehouse, separate data sets could be streamlined – like the three different data sets about the details of streets that could be combined into one authoritative source across departments. When the COVID-19 outbreak hit this city, having data already in one location enabled the Citywide Analytics Team to rapidly pull together executive information for the mayor, and as Boston’s Chief Data Officer Stefanie Costa Leabo notes, “with the infrastructure in place, we were able to spin up a dashboard for the mayor in a week with some key indicators, and then to roll that out publicly within a couple of weeks.”
Going beyond peer-to-peer horizontal data sharing and reaching vertical data sharing, the city of Boston is also using real time data feeds from the State of Massachusetts’ transit agency to gain insight that helps optimize bus routing. The state transit agency makes real time bus location data available via an API and this data is used by city transportation planners to visualize bus performance across different routes and along key city corridors. Important policy questions can be explored, such as what days of the week, times of day or particular sections of the city most need a new dedicated bus lane, or to have transit signals changed to speed overall traffic.
Example of collaborative data sharing: Integrated Public Service Data System: Charlotte-Mecklenberg, NC. In early 2020, Charlotte, NC joined its region’s Integrated Data System (IDS), and with Mecklenburg County has created a city-county database covering a wide range of public services. The existing IDS links data from 45 different social service agencies and is hosted at The Institute for Social Capital (ISC) at UNC Charlotte. The institute got its start as a workaround to the county’s challenge of not being able to share data across their own departments because of incompatible systems even within the same agencies. By having the university host the data, they were able to connect the individual level data across the various source systems.
The data is available to public and academic researchers at an individual level, so data can be linked across agencies both at a point in time and longitudinally over time. So, for example, participants in early learning programs can be traced through their time in school and beyond to see if their early literacy gains hold over the years. Homeless veterans can be tracked across various services to give a holistic picture of their needs, and their progress. Data sharing agreements are in place with all of the contributors to the database, and ISC manages the availability of that data to researchers and to the county.
In late 2020, the city plans to join the database adding police department records. The work includes a multi-disciplinary team of city and county staff ranging from police officers and teachers to data analysts and epidemiologists. Rebecca Hefner, City of Charlotte Data and Analytics Officer notes that having a specific purpose for data sharing gave a sense of focus to the city joining the region’s integrated database.
Hefner worked in the city manager’s office for years before being named as the Data and Analytics Officer and as a result, has the credibility with peers and the existing relationships across agencies to facilitate the trust needed to build data sharing agreements. As she noted, “trust takes time” and she has the benefit of having been with the city for years. She also notes that the project is moving along quickly because of the commitment of executive leaders – the city manager and county commissioner are jointly leading the violence reduction initiative.
As Hefner notes, the new city-wide enterprise data sharing agreement will cover all city data, with the exception of law enforcement sensitive data from the police department, which falls under a separate agreement. This enterprise data sharing agreement will be far more efficient than the dozens of ad hoc data sharing agreements now in place between the city and county for sharing of paying for aerial data, street segment data, parcel and property data agreements, and so on.
Conclusion. What are the factors that a government policy or data leader should consider in choosing whether to follow the government-led or the collaborative model? No two organizations or jurisdictions are alike and there is no one model that works for all situations. Deciding whether to primarily hire and train government staff for in-demand data management and analysis roles, or to engage the services of a collaborating entity are similar to the tradeoffs involved in similar decisions such as whether to buy or build software, and whether to contract out or hire government employees to provide services.
When demand for services will be uneven, with peaks and valleys of use, then the collaborative model with some level of contracting out makes more sense than having government employees go through cycles of overwork and idleness. Collaborative models also make sense when the demand from any one organization is not sufficient, but when the combined demand is sufficient to support capacity. An example of this scenario is micro mobility, where individually cities and states may not need or be able to afford to manage standards and support codebases, but their combined need for common platforms and tools has given rise to the Open Mobility Foundation, a shared resource for participating jurisdictions that provides value even to those who are not members by providing open source standards.
If demand for services will be more consistent then the focus narrows to whether the right level of internal resources can be found for the task. Building capacity in government can create long-term value and can provide for consistent high levels of innovation, as incentives are aligned toward policy mission, with Allegheny County Human Services Data Warehouse as an excellent example. When sufficient internal resources cannot be found, or if they cannot be found quickly enough, then the collaboration model can be used, either to incubate the work, or to be a permanent source of external support.
Regardless of which model is chosen, or if a hybrid of the two is used, the most important thing is to continue to place data at the center of government decision-making, so that facts and evidence can provide high quality public service that meets customer needs.
Jane Wiseman, December, 2020.
A longer version of this blog was originally posted by the IBM Center for The Business of Government at http://www.businessofgovernment.org/blog/two-models-successful-intergovernmental-data-sharing